Written by Tamar Crum, Jared E. Decker,
Robert D. Schnabel, and Jeremy F. Taylor
“My mom always said life was like a box of chocolates. You never know what you’re gonna get.” –
Forrest Gump
You may be wondering how in the world does a box of
chocolates relate to breed composition of livestock? Or, if you are anything
like me, it’s where did I hide that Halloween chocolate, I need some! I think
that there are two analogies between a box of chocolates and the breed
composition of livestock.
First, we can pick out the white chocolates and may even be
able to separate the milk chocolates from the dark chocolates. This is similar to our ability to visually evaluate
breed characteristics and sort livestock into different breed or subspecies (Bos taurus or Bos indicus influenced) based on breed characteristics. However, such visual evaluation of breed
composition is not terribly accurate.
For example, biting into a piece of dark chocolate and finding a nut when
you were expecting caramel. Crossbreeding
is an important tool in the cattle industry, as it enables us to capitalize on
breed complementarity and hybrid vigor. However,
crossbreeding complicates our ability to accurately sort animals into breeds
based on breed standard traits. Imagine a
box of chocolates that contains a few chocolates that appear to be covered in
both white and milk chocolate. Do we
sort these chocolates into the milk chocolate group or the white chocolate
group? Or, perhaps a new ‘hybrid’ group,
since neither of these groups really reflects the correct composition.
Second, a box of chocolates includes a diverse assortment of
“fillings”. The “fillings” cannot
typically be determined from just visual evaluation. The different “fillings” provide another
challenge to our being able to sort the chocolates into groups. Without reading the decoder in the box (isn’t
that cheating?) or just taking a bite out of each of them (no judgment on my
part if that is your routine!), we cannot accurately sort the chocolates. The “filling” of the chocolates is directly analogous
to the DNA of an animal. If we keep
breeding records on our animals, we can sort the animals based on their
pedigree and breed registrations. For
example, if we have offspring from a registered Angus sire and a purebred Simmental
dam, we can assume that the progeny will be 50% Angus and 50% Simmental. But, what about if we take this progeny and
breed it to a registered Angus? Will the resulting grandprogeny be 75% Angus
and 25% Simmental? Due to the random assortment of DNA (chromosomes) into the
sex cells, these proportions can vary. Not only can they differ from the 75/25 mark
in an individual, but full-sibs produced from exactly the same mating can also
be comprised of varying grandparental breed proportions. Who knew that sorting a “box of chocolates”
could be so complicated?
Understanding the breed composition of animals is a challenge,
especially for genetic researchers. Not
all members of a breed are identical. Each breed was formed by an initial
sampling of animals that were considered to be “characteristic” of a desired
breed type. Later selection and breed
development produced breeds that differed for carcass qualities, maternal
ability, or even adaptability. These characteristics make the breeds valuable
to the industry. In addition, each breed
may have different mechanisms underlying variation in traits, such as feed
efficiency or marbling. Differences in traits
between breeds reward both the producer and the consumer when crossbreeding is
used.
However, in certain genetic analyses we often need to
understand the breed composition of animals to appropriately use the data. For
example, when markers are used to generate estimates of genetic merit for
traits such as feed efficiency, the resulting prediction equations will only be
useful within the breeds that are represented in the training data set. So understanding the breed composition of the animals
will guide us in understanding how broadly useful the resulting prediction
equations will be. Because of the inaccuracies of breed identification of
crossbred animals using visual measures, a method to determine breed
composition based on sampling the “filling” will provide a more accurate
measure. We have developed an analytical
method to estimate the ancestry/breed composition of crossbred animals based on
their DNA data.
You may have heard of or even participated in the 23andMe,
Ancestry.com, and other genetic tests that are used to predict your
ancestry. You know, you used to dance in
your lederhosen until you found out that you were Scottish and so now you wear
a kilt! Think of our method as the 23andMe analysis for cows.
So how does the analysis work? In ancestry analysis, the
observed data are DNA genotypes for animals which may be full-blood, purebred,
or crossbred and the inferred factors are the ancestral or “reference”
breeds. To conduct the analysis, the
first and most important step is to determine a set of reference population
animals that genetically define the frequencies of genotypes at each tested
variant among the members of each respective breed. As you might expect, this makes it extremely
important that the breed definition for the “reference” samples is
correct. In addition to being the most
important step, determining the subset of samples that represents the diversity
within each of the “reference” breeds is technically difficult.
The reason for this is that the concept of breed and breed
membership is man-made, and has not persisted in nature. The creation of species
is a complex and lengthy process taking tens or even hundreds of thousands of
years. On the other hand, the development
of livestock breeds is a very recent concept, beginning with domestication of
cattle about 10,000 years ago and leading to the formation of herd books approximately
200 years ago. Compared to the thousands
of years cattle have roamed the earth happily mating at random, we should
probably only expect that regions of the genome with large effects on traits that define breed
characteristics have been subject to human selection and resulted in breed differences.
However, the phenomenon of drift in DNA variant frequencies over the last 200
years has caused enough differences in frequencies among breeds that we do in
fact find signal for breed identification. Our software’s output can be
represented by a figure similar to Figure 1.
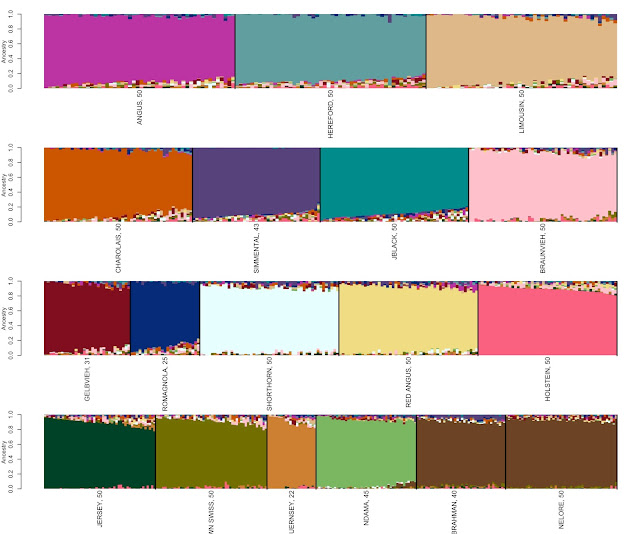 |
Figure 1: Genetic profiles for animals defined as representing 18 different breeds. Breed identification is shown below each colored block and each animal is represented as a vertical line within the figure. |
The software does not know which animals were chosen to
represent each breed but simply clusters them together based upon genetic
similarity. We then arrange the output according to the animals that were
selected to represent each breed to produce Figure 1. Each block contains a
dominant color that is representative of each breed. There also appears to be small levels of mixture
represented by the colors in the top and bottom of each block for almost all
the breeds. One interpretation of this is that there is a shortage of
statistical power to completely predict breed ancestry. However, this does not seem to vary much as
we increase the number of markers used in the analysis. So, this result could
suggest that these samples do not represent purebred animals. But, this is not
the case in the recent sense, as the animals sampled to represent each of the
breeds were traced by pedigree to ensure that they were purebred. What appears to be more likely is that this
represents breeding events that took place before the foundation animals for
each breed were selected. Two simple examples of these events are the polled
and coat color variants found in Hereford cattle. The polled mutation in Herefords is identical
to the mutation found in Angus cattle (and other Celtic breeds) and the Hereford
coat color variant is only found in white-faced European cattle (e.g.,
Simmental). This indicates that these variants, and therefore breeds, have
common, albeit, common distant ancestries.
The current software version functions to provide estimates
of the genome-wide ancestry of individuals.
Future versions may allow ancestry estimates for specific
regions of each chromosome. It provides
a method for determining the breed composition of individuals with no pedigree
information, and that were perhaps generated in a commercial environment
employing various crossbreeding systems.
Figure 2 illustrates how the genomes of crossbred animals can be separated
into components originating from their ancestral breeds, with no pedigree
information included in the analysis. By establishing the ancestry of these
individuals, we can determine cohorts for use in association studies or other
downstream analyses such as the genomic prediction of EPDs.
 |
Figure 2: Genetic profiles for 238 crossbred animals. Breed identification is shown by color and each animal is a vertical line within the figure. The key indicates which color corresponds to which ancestral breed. The animals shown are mostly Angus and Simmental. |
The goal of this research is to
develop an analytical pipeline that will enable the detection of the breed
composition of crossbred animals based on animals defined to be representative
of specific breeds. We will then use this information to enhance the analysis
of genetic and trait data. Opportunities for the use of this information are
only limited by our imagination. It was
once stated on 23andMe’s website that, “Your DNA can tell you a lot about your
family, your health, your relatives, your ancestry, your traits, and you.” (https://www.23andme.com/dna-health-ancestry/).
We hope that this software can help us do just that for cows.
Tamar Crum is a PhD student at the University of Missouri. This research is part of a study entitled
“Inference of Admixture for Cattle with Complex Ancestry”. This article was written as part of a
Walton-Berry Award given to the Decker Genomics Group at the University of
Missouri, which paid for four graduate students to attend the Beef Improvement
Federation Conference held in Athens, GA in June 2017.
Reprinted with permission from the March 2018 issue of SimTalk
.
Comments
Via Email : chiefdrlucky@gmail.com
Thank you all for reading,
God bless"